1. Brief Bio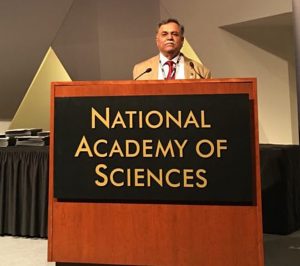
Prof. Dr. S.S. Iyengar is currently the Distinguished University Professor, Founding Director of the Discovery Lab, and Director of the US Army-funded Center of Excellence in Digital Forensics at Florida International University, Miami. He is also the Distinguished Chaired Professor at National Forensics Sciences University, Gandhinagar, India. He was awarded the 2023 Karnataka Rajyotava Award (Karnataka State’s 2nd Highest Civilian Award) on November 1st, 2023. He has been involved with research and education in high-performance intelligent systems, Data Science and Machine Learning Algorithms, Sensor Fusion, Data Mining, and Intelligent Systems. Since receiving his Ph.D. degree in 1974 from Missi. State Univ., USA, he has directed over 65 Ph.D. students, many number of postdocs, and many research undergraduate students who are now faculty at Major Universities worldwide or Scientists or Engineers at National Labs/Industries around the world. He has published more than 600 research papers, has authored/co-authored and edited 32 books, and hold various patents. Over the lifetime, his work, Brooks-Iyengar Algorithm has over 5223 publications(s) within this topic and has received 138,976 citation(s). The topic is also known as the Brooks–Iyengar hybrid algorithm. His h-index is 67 and is identified among the top 2% cited scholars and world scientists (from Stanford University and EBMs of JSAN journal. The book titled “Fundamentals of Brooks-Iyengar Distributed Sensing Algorithm” authored by Prof. Pawel (Poland) and others and published by Springer in 2020 celebrates S.S. Iyengar’s accomplishments that led to his 2019 Institute of Electrical and Electronics Engineers (IEEE) Cybermatics Congress “Test of Time Award” for his work on creating Brooks-Iyengar Algorithm and its impact in advancing modern computing. His work has been featured on the cover of many scientific journals like IEEE Transactions and the National Science Foundation’s Breakthrough Technologies report to the US Congress by his research group in both 2014 and again in 2016.
He has served on many scientific committees and panels worldwide and has served as the editor/guest editor of various IEEE and ACM journals. His books are published by MIT Press, John Wiley and Sons, CRC Press, Prentice Hall, Springer Verlag, IEEE Computer Society Press, etc. One of his books titled “Introduction to Parallel Algorithms” has been translated into Chinese. During the last thirty years Dr. Iyengar has brought in over 65 million dollars for research and education. More recently in Spring 2021, Dr. Iyengar in collaboration with HBCUs were awarded a $2.25 M funding for setting up a Digital Forensics Center of Excellence over a period of 5 years (2021-2026). He received four (4) honorary Doctor of Science, one recently from Poznan University of Technology in Poland in May 2023. He has been awarded three (3) Lifetime Achievement Awards (INTERPOL, BHU, IEEE), recently for his contribution to the field of Digital Forensics on November 8, 2022, during the 7th INTERPOL DIGITAL FORENSICS EXPERT GROUP (DFEG) MEETING at the National Forensics Sciences University, Gandhinagar, Gujarat, India. He has provided the students and faculty with a vision for active learning and collaboration at Jackson State University, Louisiana State University, Florida International University, and across the world.
Dr. Iyengar’s career is a distinguished one, marked by his incredible record of success in groundbreaking research, inspirational teaching, and excellence in community service. It is his consistent drive to fight for and promote the minority and underrepresented groups that is his passion.
Dr. Iyengar is a Member of the European Academy of Sciences, Member of the European Academy of Arts and Sciences, a Life Fellow of the Institute of Electrical and Electronics Engineers (IEEE), a Fellow of the Association of Computing Machinery (ACM), a Fellow of the American Association for the Advancement of Science (AAAS), a Fellow of the Society for Design and Process Science (SDPS), a Fellow of the National Academy of Inventors (NAI), and a Fellow of the American Institute for Medical and Biological Engineering (AIMBE). He has received various national and international awards including the crowning Test of Time Research (for his seminal work which has impacted billions of computer and internet users worldwide) and Scholarly Contribution Award from 2019 IEEE Congress on Cybermatics, the distinguished Fulbright Scientist, the Times Network NRI (Non-Resident Indian) of the Year Award for 2017, IEEE Meritorious Service award, most distinguished CVR Award at the Society for Design and Process Science (SDPS 2017), Innovation-2-Industry Award, Distinguished Rain Makers for Leadership and Research Award, World’s Best Technology Showcase award, Technology Innovation Award Louisiana Tech University Research Foundation Inventor Award, Distinguished Research Master Award, IBM Distinguished Faculty Award, and the NRI Mahatma Gandhi Pradvasi Medal at the House of Lords in London in 2013 among others. He was awarded Satish Dhawan Chaired Professorship at IISc, then Roy Paul Daniel Professorship at LSU. He has received the Distinguished Alumnus Award of the Indian Institute of Science. In 1998, he was awarded the IEEE Computer Society’s McCluskey Technical Achievement Award and is an IEEE Golden Core Member. Professor Iyengar is an IEEE Distinguished Visitor, SIAM Distinguished Lecturer, and ACM National Lecturer. In 2006, his paper entitled, A Fast-Parallel Thinning Algorithm for the Binary Image Skeletonization, was the most frequently read article in the month of January in the International Journal of High-Performance Computing Applications. His innovative work called the Brooks-Iyengar algorithm along with Professor Richard Brooks from Clemson University is applied in industries to solve real-world applications. Dr. Iyengar’s work had a big impact; in 1988, when he and his colleagues discovered “NC algorithms for Recognizing Chordal Graphs and K-trees” [IEEE Trans. on Computers 1988]. This breakthrough result led to the extension of designing fast parallel algorithms by researchers like J. Naor (Stanford), M. Naor (Berkeley), and A. A. Schaffer (AT&T Bell Labs).
His research has been funded by National Science Foundation (NSF), Defense Advanced Research Projects Agency (DARPA), Multi-University Research Initiative (MURI Program), Office of Naval Research (ONR), Department of Energy / Oak Ridge National Laboratory (DOE/ORNL), Naval Research Laboratory (NRL), National Aeronautics and Space Administration (NASA), US Army Research Office (URO), and various state agencies and companies. He has served on US National Science Foundation and National Institute of Health Panels to review proposals in various aspects of Computational Science and has been involved as an external evaluator (ABET-accreditation) for several Computer Science and Engineering Departments across the country and the world. Dr. Iyengar has also served as a research proposal evaluator for the National Academy. Dr. Iyengar has been a Visiting Professor or Scientist at Oak Ridge National Laboratory, Jet Propulsion Laboratory, Naval Research Laboratory, and has been awarded the Satish Dhawan Visiting Chaired Professorship at the Indian Institute of Science, the Homi Bhaba Visiting Chaired Professor (IGCAR), and a professorship at the University of Paris-Sorbonne.
Dr. S.S. Iyengar is an interdisciplinary computational scientist of international repute who has been a pioneer in multiple fields. Marked by his incredible record of success in the areas of world-class research, superb teaching, and excellence in community service, he has also significantly impacted industry, through his many discoveries and patents. His distinguished international and national research work have consistently been recognized by US government agencies, industry pioneers, and his research colleagues. His work has been featured on the cover of the National Science Foundation’s breakthrough technologies in both 2014 and again in 2016.
Dr. Iyengar has garnered multiple awards for his work and made fundamental contributions in a variety of areas that impact our lives today. His seminal contributions continue to be seen in places like Raytheon, Telcordia, Motorola the United States Navy, DARPA and other universities and research laboratories around the world.
HONORARY DOCTORATE OF SCIENCE TO DR. S. S. IYENGAR – Poznan University of Technology (PUT), Poznan, Poland [Link]
Poznan University of Technology (PUT), Poznan, Poland, bestowed an Honorary Doctorate of Science to Dr. S. S. Iyengar, Distinguished University Professor and Ryder Professor of the Knight Foundation School of Computing & Information Science. To formally make this announcement to FIU’s leadership, a delegation of high-ranking university officials, including Rector Professor Teofil Jesionowski, PUT, and Rector Professor Jerzy Lis, AGH University of Science and Technology (AGH UST), Cracow, Poland, who was the reviewer of the honoris causa Ph.D., visited FIU on Thursday, January 19, 2023. The formal conferral of the honorary degree took place at Poznan University of Technology in Poland on May 25, 2023.
As a globally engaged university, this prestigious recognition demonstrates Dr. Iyengar’s 5 decades of profound and enduring contributions to scholarship, science, and improved quality of life in society beyond our national borders. It acknowledges Dr. Iyengar’s scientific advancements in the area of high-performance sensor fusion, digital forensics, design and analysis of efficient algorithmic techniques for various applications and continuous collaboration with NSF, DOD agencies, universities across the world including PUT, where Dr. Iyengar served as a Fulbright Specialist in 2019. For details on his contributions, awards, students supervised and fellowships from various societies,
============================================================
- Co-invented the Cognitive Information Processing (CIM) Shell (2010), a complex event processing architecture and engine which recognizes in response to complex patterns in mission-critical, real-time applications. The CIM Shell fuses disparate data streams (text & video) to create interactive sensing-to-cognition, inspection/visualization systems providing real-time monitoring, analysis and online diagnosis of manufacturing, agricultural and oil production. CIM sends an alert, then reconfigures on-the-fly to isolate critical events and fixed failures, unlike IBM’s Watson which only identifies failures.
- Co-inventor of the FuseCPA (Brooks-Iyengar) Algorithm (1996) – About “Brooks-Iyengar Algorithm Topic” : FuseCPA (Brooks-Iyengar) algorithm is a(n) research topic. Over the lifetime, 5223 publication(s) have been published within this topic receiving 138976 citation(s).The topic is also known as: Brooks Iyengar hybrid algorithm for noise tolerant distributed control which bridges the gap between sensor fusion and Byzantine fault tolerance providing an optimal solution to the fault event disambiguation in sensor networks. It provides a computationally inspired real-time fault-tolerant solution with applications in distributed control and software reliability.
- Optimal Sensor Placement Technique (2002) for selected locations to provide protection in real-time applications. The seminal work laid the intellectual foundation for new design policies/techniques in surveillance and telecommunications.
Dr. Iyengar’s work has had a tremendous impact when applied to industries and real-world applications. In addition to the above areas, his foundational work continues to be implemented by government agencies and industry, including;
- Tactical Environmental Support System (3rd Generation—TESS(3)) Surveillance and Oceanographic Sensing (1992): Implementation of the oceanographic expert system by the Naval Research Laboratory Detachment at Stennis Space Center (NRL – SSC). This system has applications to predict oceanographic features which cannot be observed due to cloud cover in the analysis of satellite imagery.
- Sensor-Based Autonomous Mobile Robotics: Dr. Iyengar’s extensive research in the fields of robotics, sensors, and artificial intelligence has continued from his early years at Oak Ridge National Laboratory and into his current work at Discovery Lab at Florida International University. His recent work is an urban surveillance telepresence robot for use by injured police officers, demonstrating robotic technology assisting the disabled to return to work.
-
Computational Medicine: Founding Director of the Louisiana Biomedical Research Network, and development of autonomous intelligent systems for detecting radiation at Oak Ridge National Laboratory and the Jet Propulsion Laboratory, as well as discoveries in radiotherapy treatment of lung tumors, and development of an intraocular glaucoma monitoring device.
Dr. Iyengar is a member of the European Academy of Sciences, winner of the Lifetime Achievement Award from ISAM-IIT (BHU), a Fellow of the Institute of Electrical and Electronics Engineers (IEEE), a Fellow of the Association for Computing Machinery (ACM), a Fellow of the American Association for the Advancement of Science (AAAS), and a Fellow of the Society for Design and Process Science (SDPS). He was awarded the Satish Dhawan Chaired Professorship at the Indian Institute of Science (IISc), then Roy Paul Daniels Professorship at LSU. He has received the Distinguished Alumnus Award of the Indian Institute of Science. In 1998, he was awarded the IEEE Computer Society’s Technical Achievement Award and is an IEEE Golden Core Member.
One of Dr. Iyengar’s project along with Dr. Phoha(LA Tech) and Dr. Kannan(LSU) entitled “Fast Web Page Allocation on a Server Using Self-Organizing Properties of Neural Networks” was selected in World’s Best Technology Showcase in the year 2007. This is a highly competitive summit and other competitors were Los Alamos Labs, Johns Hopkins University, EPA, NASA and many other.
2. The Cognitive Information Processing (CIM) Research Tool for Predicting Rare Events from Data Sets
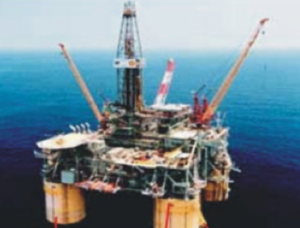
A Novel Architecture that is Changing the State-of-the-Art in Intelligent Systems
This foundational technique fuses disparate data streams, including text and video, for real-time monitoring of rare events and complex patterns of data.
Executive Summary
CIM Shell technology laid the foundation for new applications as described below.
Dr. Supratik and Dr. Iyengar are the co-inventors of the Cognitive Information Processing (CIM) shell, a system which recognizes and responds to complex patterns in mission-critical, real-time applications (see https://cacm.acm.org/news/91343-lsu-scientists-develop-new-efficiency- software/fulltext). CIM fuses disparate data streams, including text and video, to create an interactive inspection and visualization system that provides real-time monitoring, analysis, and online diagnosis for industrial applications. CIM can reconfigure itself on the fly to isolate critical events and fix failures, and underlies technologies used in manufacturing, agricultural, and oil production spaces, with notable applications including:
(1) The Online Diagnosis of Manufacturing Machines (ODMM) system, developed by SpotCheck Inc., licensed by AIlectric, and used by Novatec and ProphecySensorLytics to diagnose systems failures in factories, with a Total Addressable Market (TAM) of $9.1B in 2014, which is predicted by ABIResearch to grow to over $24.7B by 2019;
(2) The DeepSAT Video and Image Analytics application, developed by LSU and used by the NASA Ames Research Center to provide decision support for satellite missions, with a TAM of over $41B; and
(3) The DeepDrug system to shorten the developmental timeline of new drugs, developed by SynthLab, and ranked one of the top 10 systems worldwide competing for $3M in the IBM Watson AI XPrize competition. Communication of the ACM: Lsu Scientists Develop New Efficiency Software (https://cacm.acm.org/news/91343-lsu-scientists-develop-new-efficiency-software/fulltext) Story of invention
Out of those 59 teams, in the second round in 2018, only 30 teams survived. In December 2019, the DeepDrug team one of the only 10 teams surviving in the semifinals. Each of the ten teams will now get $15000 as the prize. In Feb 2020, 5 teams will be selected from these 10 to go to the final. (https://www.xprize.org/articles/30-teams-advance-in-5m-ibm-watson-ai-xprize)
Dr. Iyengar’s Team at LSU extended this framework with novel deep learning frameworks and beam search. It became the basis of the DeepDrug pipeline for drug discovery. Since 2015, his team competed in the AI XPRIZE with the DeepDrug platform. By early 2020, DeepDrug became a semifinalist in the XPRIZE (among 142 teams competing worldwide). Early 2020, COVID hit US. Mukhopadhyay used DeepDrug for discovering oral therapies for COVID 19 (Remdesivir was not an oral therapeutic). By July 2020, DeepDrug discovered a combination therapy against COVID 19. Buoyed by this success, Skymount Medical licensed the DeepDrug technology from LSU. In collaboration with Skymount Medical, the combination therapy was tested in vitro and in vivo in several laboratories where it showed effectiveness against SARS COV2. Eventually, it was approved for human trials at the Riverside University Medical Center in California. It was also approved for human trials in the UK by MHRA. In addition, it received approvals for late stage trials in both Poland and Ukraine. Currently, Skymount Medical has been valued at $1.5B by KPMG within which the valuation of the DeepDrug technology itself is around $500 million.
https://www.lsu.edu/mediacenter/news/2022/02/22deepdrug_uktrials.php
Skymount Medical, a drug discovery company using an artificial intelligence, or AI, platform developed by LSU researchers to repurpose and build new drugs, announced today that it has received approval to conduct a human clinical trial of its new oral therapeutic for COVID-19 patients in the United Kingdom.
www.lsu.edu
|
Applications and Other Links:
Article 1. SearchBusinessAnalytics.com: Cognitive computing for all? Think about it
(http://searchbusinessanalytics.techtarget.com/feature/Cognitive-computing-for-all-Think-about-it)
The hypergraph based search technique of the cognitive information management has been used for automated drug discovery. Our team at LSU is participating in the AI Xprize under the name “DeepDrug” and in the first year was ranked within the top 10 teams worldwide.
The Advocate: Baton Rouge team among Top 10 competing in $5 million IBM Watson AI XPRIZE contest (http://www.theadvocate.com/baton_rouge/news/business/article_867eb32a-da9f-11e7-a7b4-6335533ac592.html)
Greater Baton Rouge Business Report: Baton Rouge software development team advances in IBM competition for $5M
(https://www.businessreport.com/article/baton-rouge-software-development-team-advances-ibm-competition-5m)
In addition, cognitive information technology-based SpotCheck System for predictive maintenance has been licensed by AutoPredictiveCoding (Now AIlectric). J K Technosoft (part of JK Group, a $30 billion) conglomerate has signed a master services agreement with AIectric to use SpotCheck (and other technologies from AIlectric) within their own factories as well as their customers. A paper describing SpotCheck had been among the most downloaded article in the Integration Journal in June 2017. See https://www.journals.elsevier.com/integration/most-downloaded-articles.
Detailed Description
Dr. S.S. Iyengar is the co-inventor of the patented Cognitive Information Processing (CIM) Shell, a complex event processing architecture, and engine which recognizes and responds to complex patterns in mission-critical, real-time applications (IEEE Computer 2010). This new comprehensive computational framework created by Iyengar fuses disparate streaming data, like text and video, to create an interactive sensing-to-cognition, inspection, and visualization system that provides real-time monitoring and analysis that can be applied to online diagnosis of manufacturing machines, agricultural systems, and petroleum production, The CIM Shell not only sends an alert but reconfigures on the fly in order to isolate a critical event and fix the failure, unlike IBM’s Watson which only identifies the failure.
This Cognitive Information Shell was highlighted in a Business Analytics article entitled, “Cognitive computing for all Think about it”, a Business Information feature by Stephanie Neil.
The work being done with a university in India (Bidhan Chandra Krishi Viswavidyalaya, West Bengal by Dr. Biswapati Mandal) is focused on increasing specific crop productivity by using sensors to collect data on ground activity and a synthesis program to dynamically reconfigure in real time to adjust to environmental changes. The agriculture app provides actionable intelligence to scientists who use it to direct experiments and inform decision making.
Impact of the Cognitive Information Processing Shell
The CIM Shell has had a significant impact on the market. Several companies are using the process, which is licensed by AutoPredictiveCoding, LLC as noted in section 2.2. According to Spotcheck, the Total Addressable Market for this technology is predicted to be over $24.7 billion by 2019*.
• Licensed by AutoPredictiveCoding, LLC’s
• Foundational technology for Spotcheck Technology’s online Diagnosis of Manufacturing Machines
• Technology incorporated into products sold by Novattec, Prophecy, SensorLytics, and others
• Used by NASA Ames Research for satellite imagery and decision support via NVidia’s DeepSAT Video Analytics & Image program
• Used by USDA for decision support via the National Agricultural Imagery Project
(*) According to ABIresearch, https://www.abiresearch.com/press/maintenance-analytics-to-generate-247-billion-in-2/.
2.1 How Does CIM Work?
The following figure outlines how the system operates and provides trend detection rules for interpreting prior information and identifying complex events to determine associated risks and future operations.
Had the CIM Shell been integrated with the Deepwater Horizon infrastructure it would’ve interpreted prior maintenance incidents and concerns voiced by VPs and engineers as relevant events and qualified the associated risks, potentially averting the Deepwater Horizon disaster.
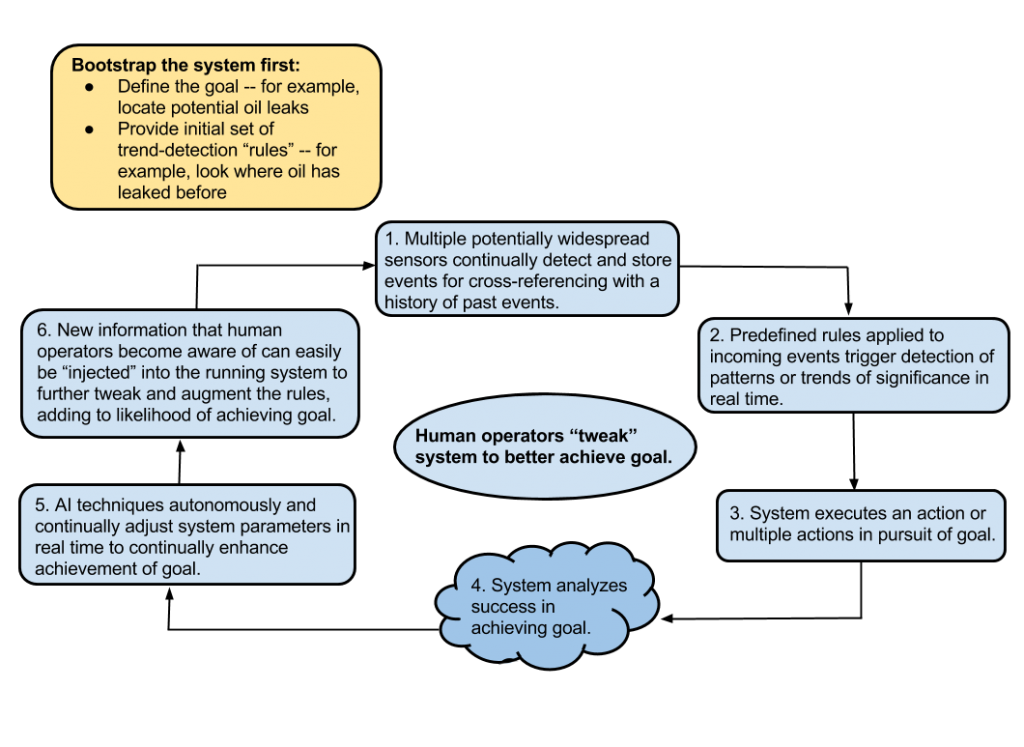
2.2 Business, Media and Academic References Demonstrating Impact and Use of CIM
SpotCheck solutions serve companies and institutions that rely on backup lines when their primary networks fail, or rely on remote, point-of-sale locations for revenue generation. SpotCheck Technology uses the system for online diagnosis of manufacturing machines. Their information and processes can be found at http://spotchecktechnology.com. Some of their clients who are also using the technology are listed below.
AutoPredictiveCoding provides automated prediction model development and analytic engines for structured and unstructured content using latest research in data science across financial services, telecom, retail and healthcare verticals. The company offers its customers turnkey Data Science solutions that are outcome based. Their Analytics Engines automate predictive model building, increase the scalability of unstructured data mining, provide sophisticated low latency pattern detection of image and video streams, and provide an out of the box integration of sensor data for verticals such as Agriculture. AutoPredictiveCoding is a current licensee of the CIM Shell technology.
Soft10’s self-learning algorithms fully automate their processes: These algorithms create, select, predict, and self-verify predictions. TEOCO leverages its expertise in ‘big data’ and ‘real-time’ capabilities to analyze and optimize network and business performance by taming the glut of telecom network, operational and business data. TreeMiner, INC is a highly scalable data mining solution using first vertical data mining solution. Zreyas Technology develops prototype solution in the Electronics and Sensor Technology domain leveraging a network of R&D partners and research labs across the US, and India.
NovaTec is an independent, owner-managed IT consulting company, which supports customers in various industries in the successful implementation of projects in the IT environment. In addition to proven services complement your own, powerful software products in the areas of performance and license management and automation of IT services, our broad product range. NovaTec is a customer for the CIM Shell technology.
Prophecy Sensorlytics affordable and patented solution lets you look into the future to predict machine malfunction and failure. Prophecy sensors are placed directly on your machines or components to automatically monitor the condition. The sensor data transmits to an easy-to-install gateway and is then sent to cloud-based servers running powerful analytic software. Results are transmitted from the server to a user-friendly app where you will view real time machine condition and maintenance advice.Prophecy SensorLytics is a customer for CIM Shell technology.
- Sensor
- Data Hub
- Router
- Cloud-based Servers
- Desktop Predictive Analytics Software
- User-friendly App
- Actionable Maintenance Advice
2.3 Total Addressable Market for the CIM Technology
ABIresearch estimates that the Total Addressable Market (TAM) for this CIM Shell technology was $9.1 billion dollars in 2014. Companies using CIM technology in this market include SpotCheck Technology for Online Diagnosis of Manufacturing Machines and currently being licensed by AutoPredictiveCoding LLC, with Novatec, and Prophecy SensorLytics customers using the technology. DeepSAT: Video Analytics and Image is using the technology for video analysis. ABIresearch predicts strong growth in both sectors for this licensed technology continuing through 2020.
Maintenance Analytics to Generate $24.7 Billion in 2019, Driven by Predictive Maintenance and Internet of Things
ABI Research forecasts that revenues from maintenance analytics will total $9.1 billion this year. Following a CAGR of 22%, the market’s size will reach $24.7 billion in 2019, driven largely by adoption of predictive analytics and M2M connectivity. While the more advanced forms of maintenance, predictive and prescriptive, still account for just 23% of this year’s market, at the end of the forecasting period they will collectively represent 60% of all revenues.
2.4 Use of the CIM Shell Technology by NVIDIA for NASA DeepSAT
DeepSAT, developed by NVIDIA provides video analytics and imaging using the CIM Shell technology. Nvidia is developing the DeepSAT technology through the NASA Ames Research Center.
“DeepSAT—A Learning Framework for Satellite Imagery” is a journal article that explains the CIM Shell technology as it applies to satellite image classification. The article appeared in 2015 in the Association for Computing Machinery (ACM) magazine. (2015 ACM. ISBN 978-1-4503-3967-4/15/11, http://dx.doi.org/10.1145/2820783.2820816.) [Basu, S., Ganguly, S., Mukhopadhyay, S., DiBiano, R., Karki, M., & Nemani, R. (2015, November). DeepSat: a learning framework for satellite imagery. In Proceedings of the 23rd SIGSPATIAL International Conference on Advances in Geographic Information Systems (p. 37). ACM.]
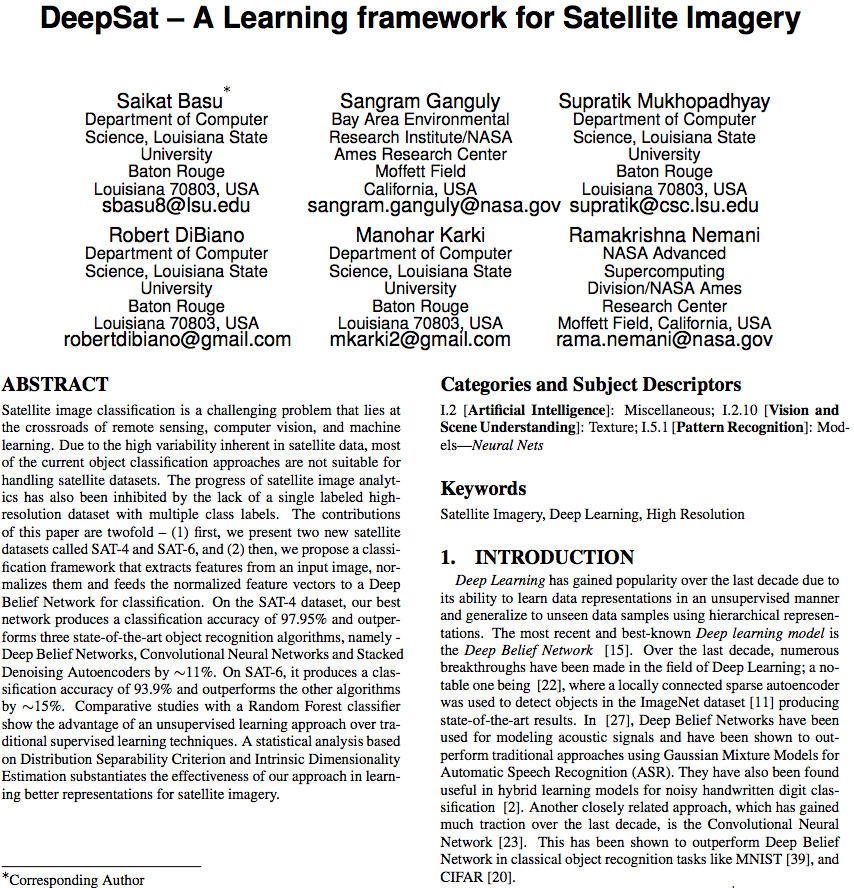
2.5 CIM shell technology used by USDA in the NAIP: National Agricultural Imagery Project
NAIP: National Agricultural Imagery Project from USDA used CIM Shell technology in conjunction with other data to provide decision support. Additional information can be found at United States Department of Agriculture Farm Service Agency and NAIP fact sheet.
National Agriculture Imagery Program (NAIP)
The National Agriculture Imagery Program (NAIP) is administered by the U.S. Department of Agriculture’s Farm Service Agency (FSA) through the Aerial Photography Field Office (APFO) in Salt Lake City, Utah. NAIP acquires aerial imagery at a resolution of 1-meter ground sample distance (GSD) for the United States during the agricultural growing season, or “leaf on” conditions. The images are orthorectified which combines the image characteristics of an aerial photograph with the georeferenced qualities of a map.
2.6 CIM shell technology additional information and applications
How CIM Shell different from IBM’s Watson?
Cognitive computing for all? Think about it article by Stephanie Neil featured in Business Information
The health care industry suffers from an ailment known as information overload. It’s a condition that makes it difficult for doctors and insurance providers to quickly determine the procedures that will be required and covered for each patient. The individual’s history, years of case files and clinical evidence must be considered -no small feat for a human. But it’s as easy as saying “aah” for Watson — IBM’s artificial intelligence computer system that processes natural language questions against a deep well of data to compute evidence-based answers in a matter of seconds. Read More >>
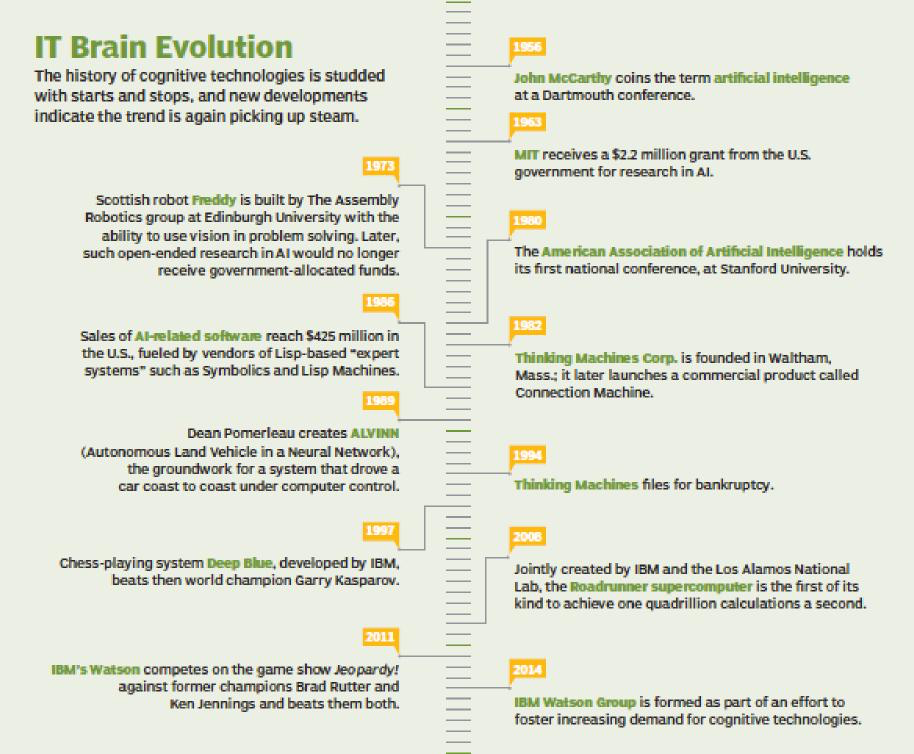
S. Iyengar, Supratik Mukhopadhyay, Christopher Steinmuller, and Xin Li “Preventing Future Oil Spills with Software-Based Event Detection“, IEEE Computer, pp: 76-78, August 2010.
Cognitive Information Management Shell (CIM Shell), a CEP system that can analyze complex events and activities and adapt rapidly to evolving situations in a wide variety of environments. By archiving past events and cross-referencing them with current events, the system can discover deep patterns and then act upon them. Agent-based techniques continually adjust CIM Shell’s parameters in near real time to adapt the system to changing environments, and human operators can easily add information to tweak the system, making goals easier to achieve. Had CIM Shell been integrated with the Deepwater Horizon infrastructure, it would have interpreted prior maintenance incidents and concerns voiced by BP’s engineers as a complex event and magnified the associated risks.
This article was featured in
For access to more content from the IEEE Computer Society,
see computingnow.computer.org.
3. The FuseCPA (Brooks-Iyengar) Algorithm
The Profound Contribution of the FuseCPA (Brooks-Iyengar) Algorithm
FuseCPA/(BI) framework guarantees in terms of Consensus, Precision, and Accuracy in the presence of both noise and deliberate deception in building a fault-tolerant distributed sensor network.
World Renowned FuseCPA (Brooks – Iyengar) Distributed Sensing Algorithm
About “Brooks-Iyengar Algorithm Topic” : FuseCPA (Brooks-Iyengar) algorithm is a(n) research topic. Over the lifetime, 5223 publication(s) have been published within this topic receiving 138976 citation(s). The topic is also known as: Brooks–Iyengar hybrid algorithm.
The Brooks–Iyengar hybrid algorithm for distributed control in the presence of noisy data combines Byzantine agreement with sensor fusion. It bridges the gap between sensor fusion and Byzantine fault tolerance. This seminal algorithm unified these disparate fields for the first time. Essentially, it combines Dolev’s algorithm for approximate agreement with Mahaney and Schneider’s fast convergence algorithm (FCA). The algorithm assumes N processing elements (PEs), t of which are faulty and can behave maliciously. It takes as input either real values with inherent inaccuracy or noise (which can be unknown), or a real value with apriori defined uncertainty, or an interval. The output of the algorithm is a real value with an explicitly specified accuracy. The algorithm runs in O(NlogN) where N is the number of PEs: see Big O notation. It is possible to modify this algorithm to correspond to Crusader’s Convergence Algorithm (CCA), however, the bandwidth requirement will also increase. The algorithm has applications in distributed control, software reliability, and High-performance computing. Wikipedia
IMPACT of BROOKS – IYENGAR ALGORITHM for AGRICULTURE IN INDIA
Brooks – Iyengar Technique has been used extensively in the field of agriculture in India. The details are as follows:
Dr. Iyengar, serving as the Chief Scientific Advisor at Xiphias Software Technologies in Bangalore, has recently secured a patent for the innovative concept of ‘Kiosk for Automated Soil Testing’. The research conducted by the collaborators, including Dr. Leena, received funding from Gandhi Krishi Vigyana Kendra (GKVK) in Bangalore, India. The pivotal component of this cutting-edge system is the Brooks-Iyengar Algorithm, which has been developed to revolutionize the process of analyzing soil composition.
Under Dr. Iyengar’s leadership, Xiphias Software Technologies has made significant strides in the field of agricultural technology by introducing an advanced and automated approach to soil testing. The recently patented system not only streamlines the traditionally labor-intensive soil testing procedures but also enhances accuracy and efficiency through the incorporation of the Brooks-Iyengar Algorithm.
The Brooks-Iyengar Algorithm, developed by Dr. Iyengar, serves as the backbone of the entire soil testing system. Its intricate design and intelligent processing capabilities enable the software to analyze soil samples with a high level of precision. This breakthrough technology is poised to redefine the agricultural industry’s approach to soil analysis, providing farmers and researchers with a reliable and rapid solution for assessing soil quality and composition.
More Details can be found at: https://www.xiphiastec.com/iso-certifications.html
As the Chief Scientific Advisor, Dr. Iyengar’s expertise and dedication have played a pivotal role in the successful development and implementation of the Automated Soil Testing system. This innovative technology not only showcases Xiphias Software Technologies’ commitment to pushing the boundaries of scientific exploration but also underscores Dr. Iyengar’s contribution to advancing agricultural practices through the integration of sophisticated algorithms and automation. The patent secured for Automated Soil Testing stands as a testament to the company’s commitment to excellence and its continuous pursuit of groundbreaking solutions in the realm of agricultural technology.
Application in DARPAS’s program demonstration with BBN, Cambridge Massachusetts, MURI, researchers from PSU/ARL, Duke, University of Wisconsin, UCLA, Cornell, and LSU.
In 2000, the DARPA program manager used two major demonstrations to showcase SensIT’s advances and document the ability of sensor networks to provide new capabilities. One
demonstration took place at the Twentynine Palms, California Marine Training grounds in
August 2000, the other took place at BBN offices in Cambridge, Massachusetts in October 2011. Dr. Gail Mitchell of BBN coordinated this work for BBN, DARPA’s SensIT integration contractor. Both demonstrations used the FuseCPA (Brooks-Iyengar) fusion approach to combine sensor readings in real-time. Acoustic, seismic, and motion detection readings from multiple sensors were combined and fed into a distributed tracking system. The first deployment was effective but noisy. The second demonstration built on the success of the first testing California. An improved outfielder algorithm was used to determine which node was best situated to continue existing tracks. This work was an essential precursor to the Emergent Sensor Plexus MURI from Penn State Applied Research Laboratory (PSU/ARL) with Dr. Sashi Phoha as PI. In that MURI, researchers from PSU/ARL, Duke, University of Wisconsin, UCLA, Cornell, and LSU extended SensIT’s advances to create a practical and survivable sensor network applications.
- Unified the fields of Fault Tolerance and Sensor Fusion—revolutionary contribution!
- Incorporated by DARPA into SensIT technology and its demonstrated advances.
- Allowed combine sensor readings in real-time
- Combined acoustic, seismic, and motion detection readings from multiple sensors;
- Fed into a distributed tracking system
- Incorporated into modern-day LINUX & Android OS
- Combines data in fault tolerance
- Used by 99% of the world’s top supercomputers
- 79% of all smartphones worldwide
- 100% of users accessing the Internet
3.1 Business, Media and Academic References Demonstrating Impact and Use of the FuseCPA (Brooks-Iyengar) Algorithm
IMPACT ON HEALTH SUPPORT
Application of this algorithm in modern day Linux operating system for combining data in fault-tolerance.(MINIX)
In 1996, Iyengar’s group, in collaboration with Brooks and with funding from Oak Ridge National Laboratory and the Office of Naval Research, invented a method of fault tolerance modeling that offers a computationally inspired real-time management solution. This work has emerged in new versions of real-time extensions to Linux Operating Systems. Many of these algorithms were used and installed in the RT Linux Operating System. They are now working on formal model verification by incorporating the algorithms into a new embedded kernel for robotic applications.
The profound contribution of the Brooks-Iyengar Distributed Computational Sensing work has enhanced new real-time features by adding fault tolerant capabilities.
V. Kumar, “Impact of Brooks-Iyengar DistributedSensing Algorithm on Real Time Systems,” in IEEE Transactions on Parallel and Distributed Systems, vol. 25, no. 5, pp. 1370-1370, May 2014.
In this paper we evaluate the merit of Brooks-Iyengar distributed sensing algorithm and highlight its impact on cost-effective processing of real-time sensor data stream. We discover that it has a long-lasting impact, not only on sensor networks, but also on computer operating systems. We believe that as we learn more about the usefulness of this algorithm, it will become an essential component of systems that use sensors in some form.
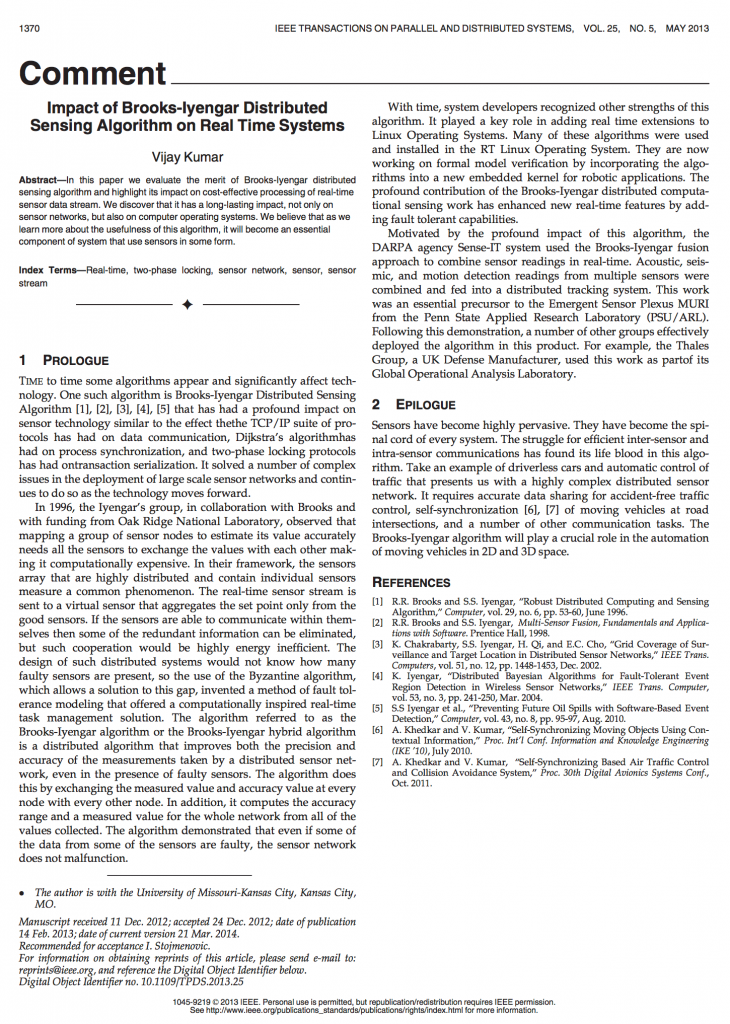
Buke Ao, “Robust Fault Tolerant Rail Door State Monitoring Systems: Applying the Brooks-Iyengar Sensing Algorithm to Transportation Applications,” in International Journal of Next-Generation Computing, Vol. 6, No. 3, November 2015.
In a moving train, the acceleration and deceleration adversely effect the sensor systems, which may induce errors into the system. Today’s technology does not guarantee success and safety in all situations. There are two important situations that are critical for the safety of passengers when embarking and disembarking. Distributed sensing networks needed to control the train doors require a fusion of the sensor inputs to provide accurate automatic opening and closing with minimum traction. Brooks-Iyengar Distributed Sensing algorithm can be used to provide a fault tolerant automatic sensing platform for closing doors based on the following scenario.
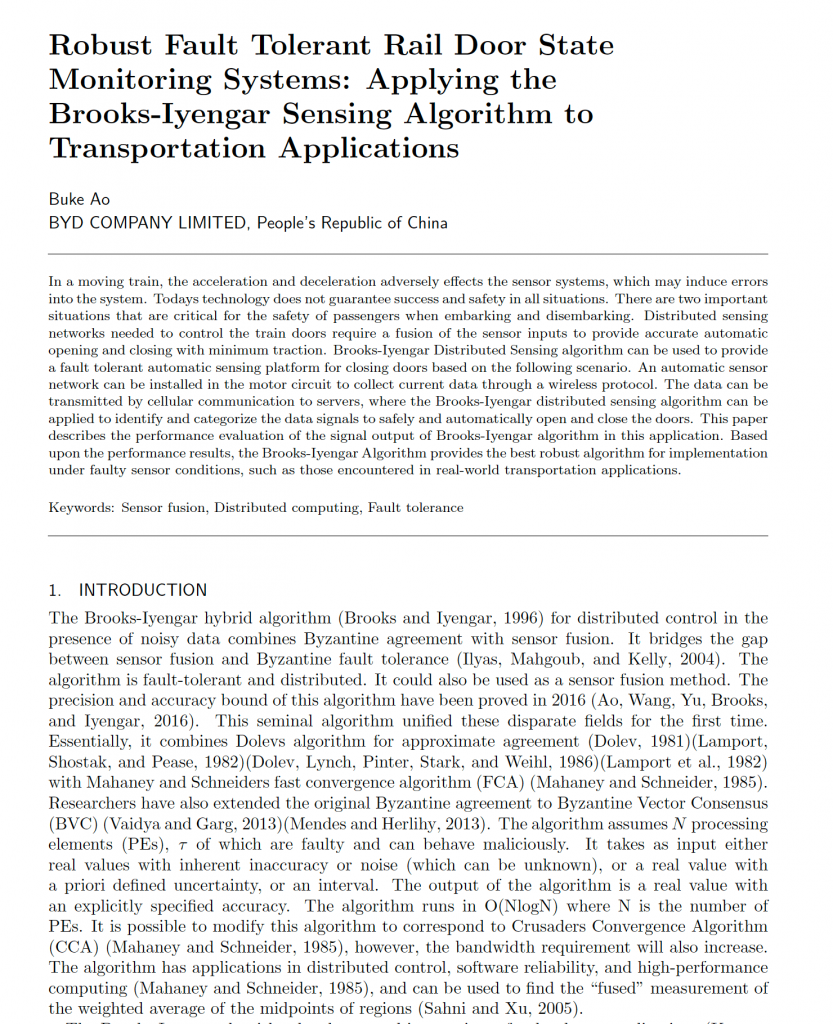
Application of FuseCPA (Brooks-Iyengar) Algorithm for Social Networks Fusions
The task of identifying and tracking influential nodes in the ever-growing information networks is crucial for many real-world applications that require information propagation (e.g., viral marketing). Although existing studies have been focusing on identifying influential nodes in social networks, most of them have focused on networks with homogeneous nodes (e.g., user-user nodes) and have also ignored the impact of relevant contexts (e.g., social, temporal, and spatial). The contextually enriched information networks have heterogeneous entities that are interconnected and complement each other’s functionality. Hence, the classical techniques popular in modeling the spreading of information in simple networks may not be efficient. An interesting example is the exploitation of social networks to identify the influential candidate in the U.S. presidential election. For the 2016 election, several models predicted about 80% winning chances of Hillary Clinton. Most of those studies exploited user polls, activities and posts from different social networks to capture the correlation between different parameters and to formulate the influence propagation for both candidates, and predicted the winning likelihood using their influence scores. As the correlation need not necessarily imply causation, the actual result was the opposite of the predictions. This implies that those models should have been impacted by some noisy information from the social networks. For instance, for any two users, having posts in support of one candidate need not necessarily indicate their voting decision. From this example, we can argue that a contextual exploitation of information on social networks is essential to model the influential parameters and to handle the noisy information.
In this study, we propose a model called MITS (Multi-context Influence Tracking on Social Network) represents the contextual exploitation of heterogeneous nodes (i.e. user-location nodes in Location-based Social Networks (LBSN)), formulates the locality-aware spatial-socio-temporal influence tracking problem using FuseCPA (Brooks-Iyengar) hybrid algorithm, and uses the geo-tagged check-in data to identify and track the locality influence. The empirical evaluation of the proposed model on two real-world datasets, using the Susceptible-Infected-Recovered (SIR) epidemic technique, coverage, and ratio of affection metrics demonstrate a significant performance gain (e.g., 10% to 85% on coverage and 14% to 39% on ratio of affection) of the proposed model against other popular techniques, such as degree centrality, betweenness centrality, closeness centrality, and PageRank.
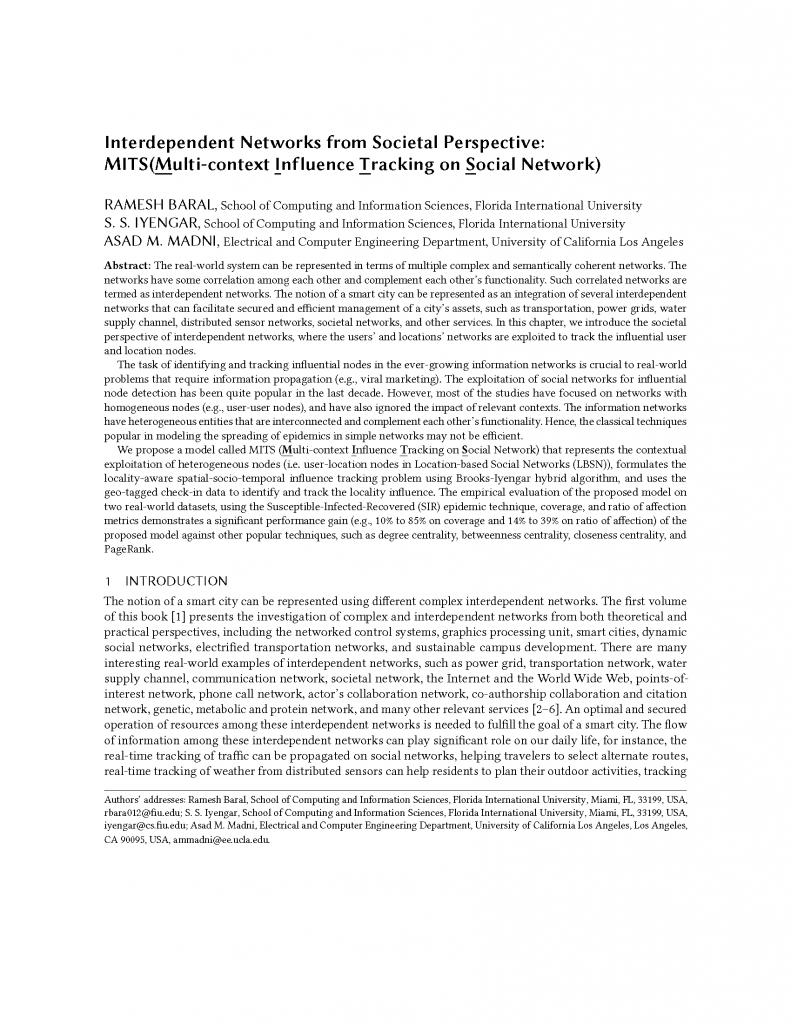
Click here to view full document.
Sustainable Interdependent Networks II
– From Smart Power Grids to Intelligent Transportation Networks
Impacts of FuseCPA (Brooks-Iyengar) Algorithm on Ph.D. Dissertations
More than 28 dissertations from highly ranked universities (e.g. MIT, Carnegie Melon University, RICE University, University of Southern California, and University of Pennsylvania) have extensively inspired by the FuseCPA (Brooks-Iyengar) Algorithm or applied it in their dissertation.
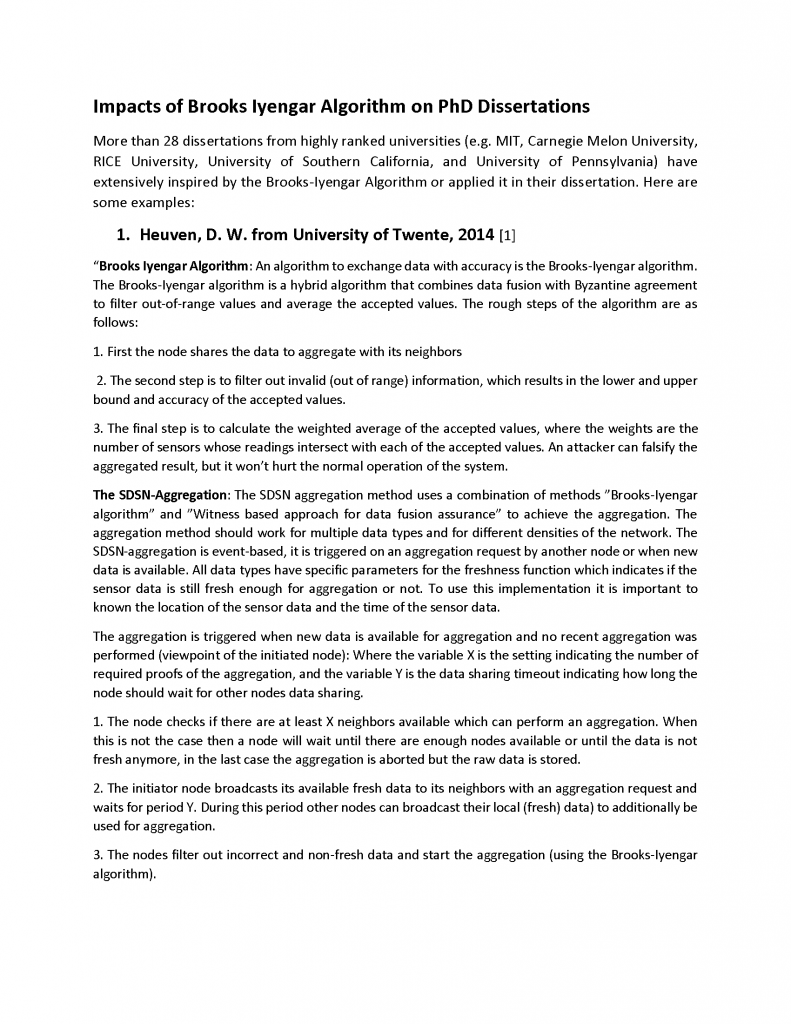
3.2 Select Letters
Carleton University is developing a formal model verification by incorporating the algorithms into a new embedded kernel for robotic applications.
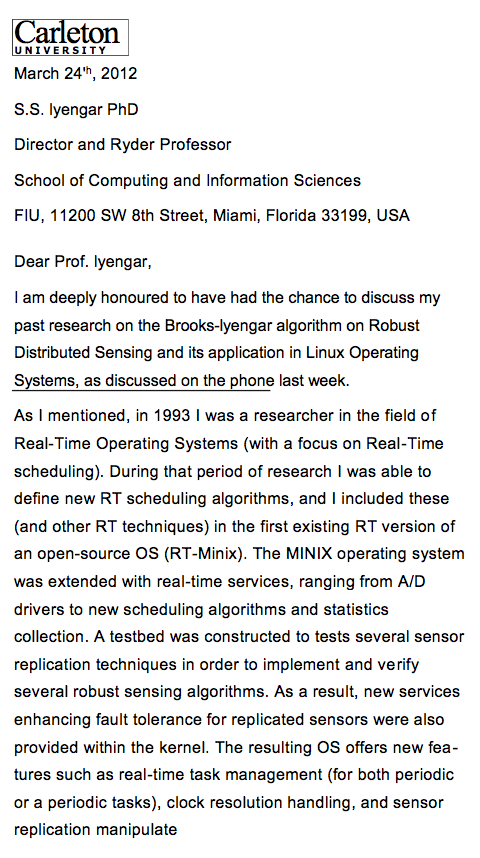
…the impact of this work has been significant. The Brooks-Iyengar Algorithm makes it possible to extract reliable data from sensor networks when some sensors are unreliable, thus adding a fault tolerant aspect. Unreliable sensor data are typically the case in systems that Raytheon develops for its customers, so applications of the algorithm made Raytheon and its customers more successful without increased investment in improved sensor reliability. This saved money, always important in today’s increasingly budget conscious environment.
…the Brooks-Iyengar algorithm continues to have significant impact where it is applied to Raytheon’s programs.~ Intelligence, Information and Service Raytheon
Citation from Raytheon Company
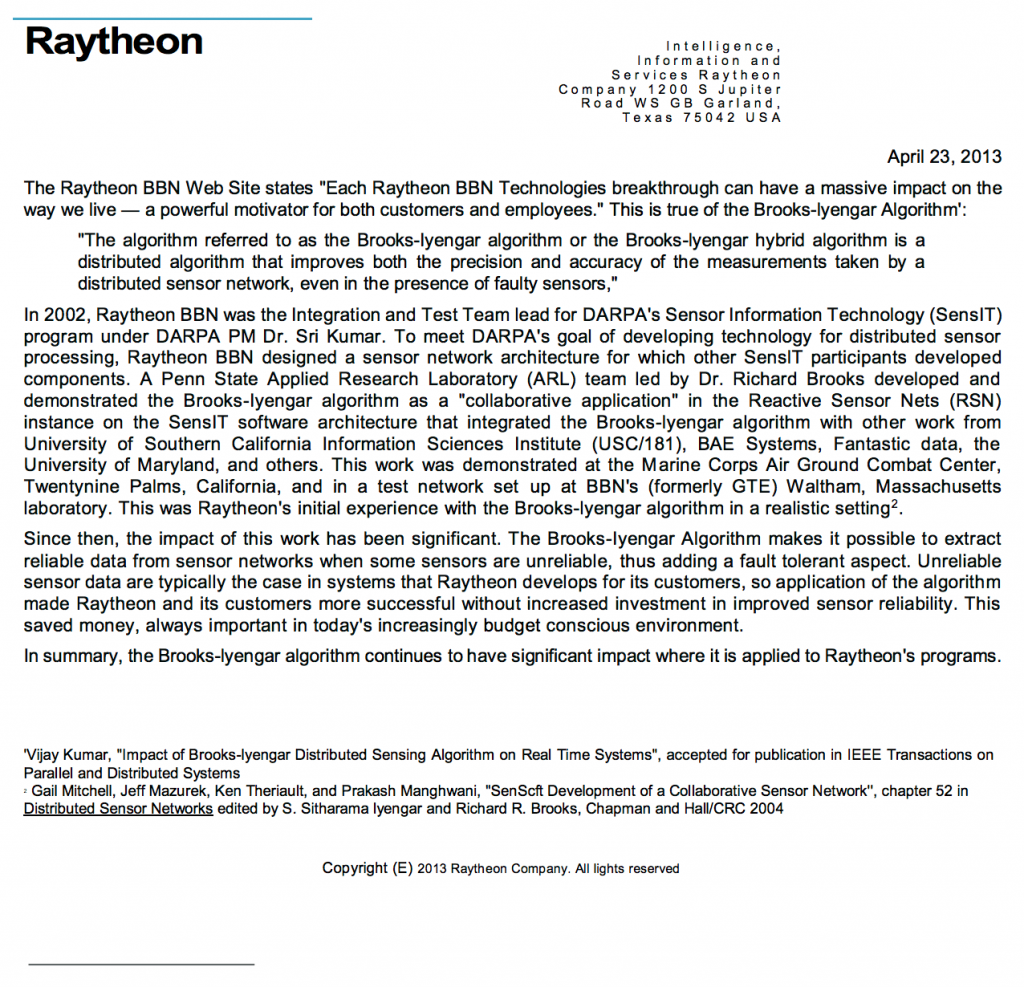
Telcordia Technologies, Inc., also incorporated the Brooks – Iyengar Algorithm into their work.
…The seminal work of professor Iyengar and his colleagues on sensor networks infrastructure constitutes a foundation for sensor placement and optimization of information obtained from sensor networks. The ideas and algorithms introduced by Iyengar et al. Played an important role in Telcordia patent 019576 entitled “Method for placement of sensors for Surveillance” and Motorola patent 7688793 entitled, “Wireless sensor node group affiliation method and apparatus,” both of which build up upon his pioneering work.
I believe that professor Iyengar work on sensor deployment, coverage, and surveillance has led to major advances in network technologies….~ Marek Rusinkiewicz Vice President for research and General Manager of Telcordia
CITATION FROM TELCORDIA TECHNOLOGIES INC.
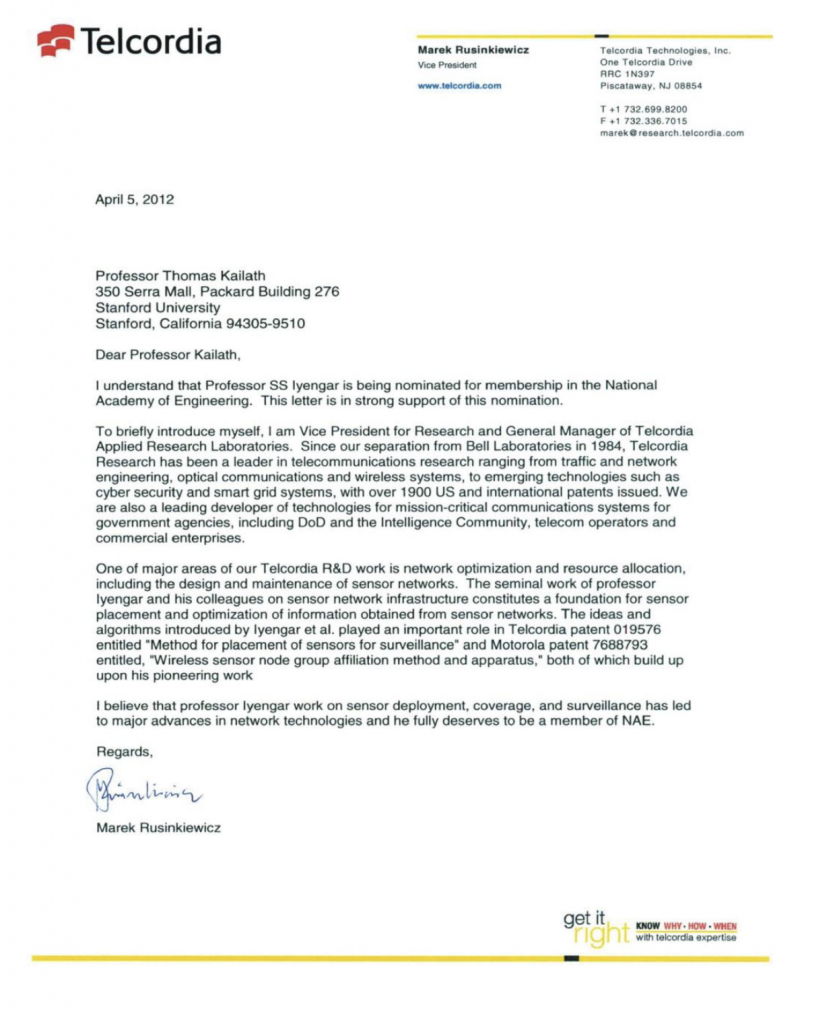
3.3 The FuseCPA (Brooks-Iyengar) Algorithm Demonstrates Potential for Millions of Dollars of Future Market Growth
Iyengar’s work has had a significant impact in industrial applications/ research, and continuing scientific research. Many new ideas have been spawned from his seminal work, resulting in a variety of new innovations by industries such as Motorola, Telcordia, Boeing, and even new chip-design at universities. New applications from industrial research are ongoing, each resulting in millions of dollars of market growth.
The intellectual advancement of his ideas has been incorporated into over 23 Ph.D. thesis and other new thesis and dissertations resulting in scientific discoveries around the world. His work has impacted thesis in Germany, the United States, and China. His foundational work has also resulted in innumerable funding opportunities provided by the National Science Foundation to continue to advance research in this significant area.
4. The Optimal Sensor Placement Technique
The Seminal Contribution of the Optimal Sensor Placement Technique
Co-inventor of an optimal sensor placement technique relating to a limited number of sensors at selected locations in order to provide protection to all locations in real-time applications, which has been included in patients by Telcordia and Motorola, as well as other companies. (IEEE Computers, 2002). This groundbreaking work has laid an intellectual foundation for designing policies and techniques in many areas of real-time applications from surveillance systems to telecommunications systems.
4.1 Optimal Sensor Placement Technique Impact on Industry
Iyengar’s work has had a significant impact in industrial applications/ research, and continuing scientific research. Many new ideas have been spawned from his seminal work, resulting in a variety of new innovations by Telcordia, Boeing, and even new chip-design at universities. New applications from industrial research are ongoing, each resulting in millions of dollars of market growth.
The intellectual advancement of his ideas has been incorporated into over 23 Ph.D. thesis and other new thesis and dissertations resulting in scientific discoveries around the world. His work has impacted thesis in Germany, the United States, and China. His foundational work has also resulted in innumerable funding opportunities provided by the National Science Foundation to continue to advance research in this significant area.
4.2 Business, Media and Academic References Demonstrating Impact and Use of the Optimal Sensor Placement Technique
Telcordia Technologies, Inc., incorporated the Optimal Sensor Placement Technique into their work.
Review reference letter from Telcordia Technologies, Inc.
Millions of dollars of market growth. Iyengar’s work has had a significant impact in industrial applications/ research, and continuing scientific research.New applications from industrial research are ongoing, each resulting in millions of dollars of market growth.
“Grid coverage for surveillance and target location in distributed sensor networks,”
K Chakrabarty, SS Iyengar, H Qi, E Cho, “Grid coverage for surveillance and target location in distributed sensor networks,” IEEE Transactions on Computers 51 (12), 1448-1453
“Distributed Bayesian algorithms for fault-tolerant event region detection in wireless sensor networks,” appeared in IEEE Transactions on Computers 53 (3), 241-250, and further explains the groundbreaking Optimal Sensor Placement technology.
4.2.1 Highly Cited Papers
The precise location of a stationary target and for a moving target its trajectory of motion are of prime importance in many fields such as surveillance or environmental monitoring. Before 2001, most prior work focused exclusively on efficient sensor communication and sensor fusion for locating a target and had solutions through optimal placement of sensors (for target location). In a now highly cited paper (more than 1000 citations on Google Scholar), Dr. Iyengar (and his team) provided an elegant integer linear programming solution for minimizing the cost of sensors for complete coverage in a 2D and 3D grid field. By formulating the problem as a cost minimization under coverage constraints, he leveraged efficient ILP solvers for combinatorial optimization problems. His solution simplified target location considerably by developing placement strategies leveraging ILP and the theoretical framework of identifying codes to determine the best placement of sensors so that every grid point in the sensor field is covered by a unique subset of sensors. In this way, the set of sensors reporting a target at a given time uniquely identifies the grid location for the target. The determination of the trajectory of a moving target follows in a similar fashion from time series data.
1433 citations: Sensor deployment and target localization based on virtual forces – Y Zou, K Chakrabarty, IEEE INFOCOM, 2003
702 citations: Sensor deployment and target localization in distributed sensor networks – Y Zou, K Chakrabarty, ACM Transactions on Embedded Computing Systems, 2004
1251 citations: Grid coverage for surveillance and target location in distributed sensor networks – K Chakrabarty, SS Iyengar, H Qi, E Cho, IEEE Transactions on Computers, 2002
863 citations: Sensor placement for effective coverage and surveillance in distributed sensor networks – SS Dhillon, K Chakrabarty, Wireless Communications and Networking, 2003
470 citations: Sensor placement for grid coverage under imprecise detections – SS Dhillon, K Chakrabarty, SS Iyengar, Information Fusion, 2002
4.2.2 Optimal Sensor Placement Technique Industry Impact: Patents
8019576: Method for placement of sensors for surveillance – Issued: September 13, 2011, Assignee company: Telcordia.
7688793: Wireless sensor node group affiliation method and apparatus – Issued: March 30, 2010, Assignee company: Motorola.
7676805 B2: Wireless sensor node executable code request facilitation method and apparatus – Issued: March 9, 2010, Assignee company: Motorola.
8131839 B2: Method and apparatus for resource assignment in a sensor network – Issued: March 6, 2012, Assignee company: Motorola.
WO 2015132691 A2: Method for deploying sensors – September 11, 2015.
US 7480395 B2: Decentralized detection, localization, and tracking utilizing distributed sensors – Issued: January 2009.
US 7091902 B2 (Xerox Corporation): Systems and methods for characterizing the coverage of ad hoc sensor networks – Issued: August 2006.
4.2.3 Optical Sensor Placement Technique Documented Impact on Research at Boeing Corporation
Mattikalli, R. Fresnedo, R. Frank, P. Locke, S. Thunemann, Z. Optimal Sensor Selection and Placement for Perimeter Defense, 2007.
4.2.4 Documented Impact on Research at Department Of Defense
Capt. S. Hynes and N. S. Rowe, “Multi-Agent Simulation for Assessing Massive Sensor Deployment”, Article at Naval Postgraduate School, 2004
We present the design and implementation of a multi-agent simulation that models deployment and coverage of sensors performing collaborative target detection. The focus is on sensor networks with enough sensors that humans cannot individually manage each. Experiments evaluated both known and novel deployment algorithms, and considered effects of the sensor type, number of sensors deployed, presence of obstacles, and mobility of the sensors.
4.2.5 Impact on Graduate Theses and in Diverse Areas (Including International Impact)
- Integrated Circuit Design: On-Chip Thermal Sensor Placement, M.S. Thesis at UMass-Amherst (Yun Xiang), 2008
- Wireless Networks: Secure Localization and Node Placement Strategies for Wireless Networks, Ph.D. Thesis at Auburn University (Santosh Pandey), 2007
Robotics: Energy-Efficient Mobile Robots, Ph.D. Thesis (Yongguo Mei) at Purdue University (2007)
- Sensor Management: Global Sensor Management: Allocation of Military Surveillance Assets, Ph.D. Thesis at NC State University (Kristin Arney), 2008
- Wireless Sensor Networks: Effiziente Kommunikation und Optimierung der Knoten-Positionierung in drahtlosen Sensornetzen unter Ausnutzung räumlicher Korrelationen (in German), Ph.D. Thesis at Technical University of Aachen, Germany (Frank Odewurtel), 2011
- Distributed Self-Deployment in Visual Sensor Networks,(MS thesis), Christopher Allan Beall August 2006, The University of Tennessee, Knoxville.
- Energy-efficient coverage with wireless sensors(Ph.D. thesis), Guanqun Yang, Iowa State University,2010
- Intelligent deployment strategies for passive underwater sensor networks, Ph.D. thesis), Rochester Institute of Technology, Erik Golen,2009,
- Collaborative Area Monitoring Using Wireless Sensor Networks with Stationary and Mobile Nodes, Theofanis P. Lambrou, Ph.D. thesis, University Of Cyprus.
- Self-Organizing Hybrid Sensor System with Distributed Data Fusion For Intruder Tracking and Surveillance, RAVISHANKAR PALANIAPPAN M.S. University of Central Florida, 2003
- Collaborative Solutions to Visual Sensor Networks, Mahmut Karakaya, (Ph.D. Thesis), The University of Tennessee, Knoxville,2011.
- Cargo Monitoring: Optimal Communications Systems and Network Design for Cargo Monitoring, Ph.D. Thesis at University of Kansas (Daniel Fokum),
- An Energy-Efficient Distributed Algorithm for k-Coverage Problem in Wireless Sensor Networks, Chinh Trung Vu,(MS thesis), Georgia State University,2007.
- MULTI-AGENT SIMULATIONS (MAS) FOR ASSESSING MASSIVE SENSOR COVERAGE AND DEPLOYMENT, Sean E. Hynes, (MS thesis), Naval Postgraduate School, 2003.
- Human Activities Space: A Multi-Sensor System for a Human Activities Space Aspects of Planning and Quality Measurement, Blekinge Institute of Technology, Sweden, Licentiate Dissertation Series, 2008.
- Structural Health Monitoring: Identification of Damage Using Lamb Waves, Springer Book Volume 2009.
- Dimensional Measurement: Distributed Large-Scale Dimensional Metrology: New Insights (book).
In the area of sensor networks narrowly defined, the citation below used in over 23 Ph.D. thesis.
Chakrabarty, K., S. S. Iyengar, H. Qi, and E. Cho. 2002. “Grid Coverage for Surveillance and Target Location in Distributed Sensor Networks.” IEEE Transactions on Computers 51(2):1448-1453.
4.2.6 Impact on National Science Foundation (NSF) Grants
Several NSF grants were awarded to researchers who have built upon and leveraged the pioneering work on sensor deployment and minimalistic sensor networks. Here is a snapshot of some these grants:
Award Number: CNS-1054935 (“CAREER: A Theoretical Foundation for Achieving Sustainability and Scalability in 3D Wireless Sensor Network Deployments”)
Award Number: CNS- 1152134 (“Optimal Surface Gateway Deployment for Underwater Acoustic Sensor Networks”)
Award Number: 0449309 (“Collaborative Signal and Information Processing in Sensor Networks”)
Award Number: CNS-1149611 (“SensorFly: Minimalistic Dynamic Sensing and Organization in Groups of Semi-Controllable Mobile Sensing Devices”)
5. Tactical Environmental Support System (Surveillance and Oceanographic Sensing)
In the fields of surveillance and oceanographic sensing, Dr. Iyengar’s impact has been enormous. His foundational research provided the basis for a much larger effort involving U.S. Navy laboratories, industry partners, and leading universities to construct next-generation U.S. Navy surveillance systems. His work was the centerpiece of the Navy’s pioneering efforts in developing computerized image analysis system leading to the first fully automated U.S. Navy system for interpretation of satellite images of the ocean. his work on image analysis is the fundamental framework for today’s operational U.S. Navy systems. Expanding the frontiers of image analysis science, and are captured in his book, Advances in Distributed Sensor Integration: Applications in Theory, published in 1995 and his new, soon to be published work, The Design and Analysis of Algorithms for Processing Digital Satellite IR images.
5.1 Contributions to U.S. Navy
Dr. Iyengar’s research on image processing systems was used in 1988-93 by the U.S. Navy as a centerpiece of architecture. Dr. Iyengar addressed the problem of linking of low-level features of oceanographic objects. Without useful result in this key area, the work of other laboratories involved with this project could not have been integrated into a working prototype system. In three years Dr. Iyengar’s research served well as the centerpiece of his pioneering efforts in computerized image analysis systems.
5.3 Select Letters
…Prof. Iyengar’s use of non-linear probabilistic relaxation to perform feature labeling was innovative and well executed. His work was a key factor leading to the first fully automated interpretation of a satellite image of the ocean in 1989…
~ Ronald Holyer Head, Computer Sciences Section, US Naval Research Office
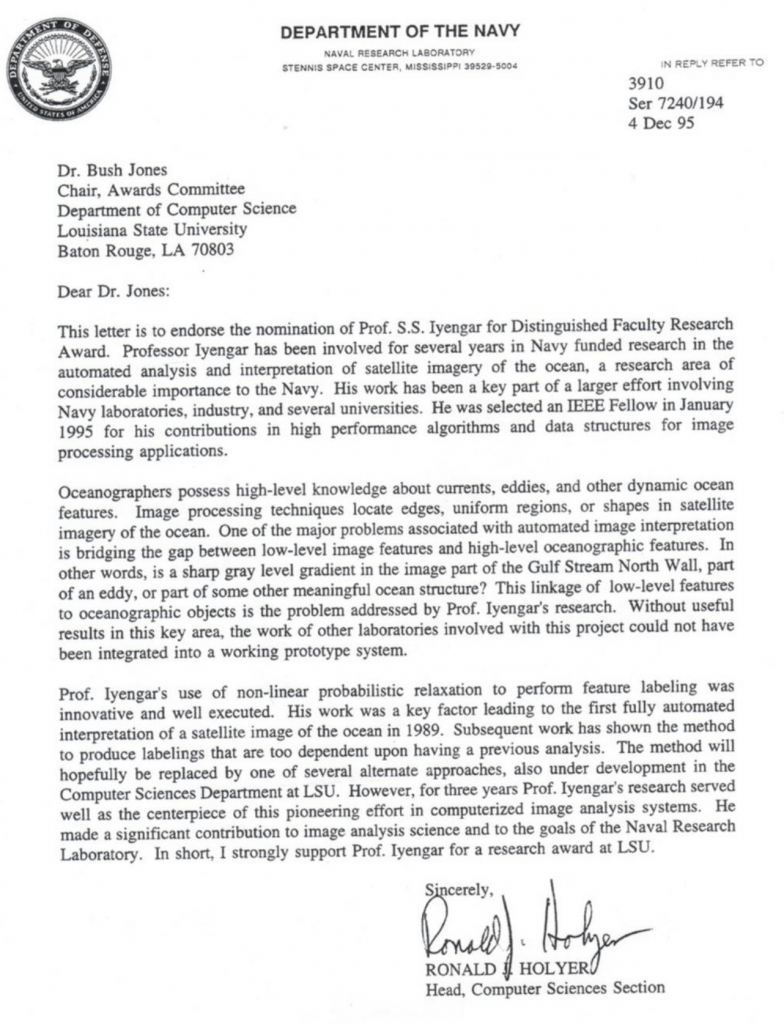
The Naval Research Laboratory (NRL-SSC) has developed an expert system with a rule base that “knows” about the evolution of mesoscale features in the Gulf Stream region of the North Atlantic. The expert system uses rules that describe the features’ kinematics to evolve an earlier environment. Hence,it is proposed as a candidate for implementation on the Tactical Environmental Support System, third generation (TESS(3)).
Read the full documentation on Oceanographic Expert System: Potential for TESS(3) Applications
6. Contributions to Sensor-Based Autonomous Mobile Robotics: Theory, Research, and Impact
Jointly with Department of Energy Oak Ridge National Laboratory
During the last four decades, Professor Iyengar has been laying a foundational approach by combining the deep mathematical insights into algorithmic foundations with an equally deep view of practical considerations in many areas. Robotics is one of the areas that benefited from his keen insights and solid, rigorous and elegant solutions, which has had an impact in technological advances and for real-world applications. This impact of his contributions can be documented both in industrial applications with a research focus. Translation of his work into the applications and its commercial impact has been significant.
Very few mobile robot manufacturing companies existed in the 1980s in the U.S. (or elsewhere), and indeed, federal agencies had to seed in-house efforts for building mobile robots needed for their specific mission needs. U.S. Department of Energy (DOE) launched an initiative at Oak Ridge National Laboratory (ORNL) to build mobile intelligent robots for autonomous operation in radiation-prone environments, which could potentially be too dangerous for human operations. Dr. Iyengar, jointly with Oak Ridge National Laboratory researchers, started the development of these intelligent robots (HERMES I, II, and II series of experimental robots) in which his invention in designing real-time algorithms for mapping terrain to facilitate the monitoring and processing of asynchronous information.
Furthermore, the enhanced conflict resolution strategies and rule incorruptibility features of the asynchronous production systems for monitoring the autonomous robot in a real-time environment were all a part of his invention and discovery on the HERMES series robots. His contributions represent a significant increase in research capabilities at ORNL-CESAR robotic research. His impact and contribution in HERMES at the Center for Engineering Systems for advanced Research at ORNL represents a foundational increase in research capabilities for the DoD CESAR Laboratory.
His innovations and algorithms developed are currently preserved at the Archive Facilities at ORNL.
Dr. Iyengar’s research in the fields of robotics, sensors, and artificial intelligence has continued from his early years at Oak Ridge National Laboratory and into his current work at Discovery Lab at Florida International University, where he is working on the new future of law enforcement in the form of a telepresence robot for use by injured police officers to maintain their presence on city streets and within the law enforcement career field, when they may otherwise have been medically retired.
6.1 History at Oak Ridge National Laboratory (ORNL) Robotics
In the early 1980’s the U.S. Department of Energy (DOE) launched an initiative at Oak Ridge National Laboratory (ORNL) to build mobile robots for autonomous operation in radiation-prone environments, which could potentially be too dangerous for human operations. As the then electro-mechanical devices were being built at ORNL, it became apparent that sophisticated navigation systems were essential for the success of their missions. The problem was further compounded by the unstructured DOE environments, e.g., after an incident, and the limited reliability of sensors, namely, ultrasonic and low-resolution cameras. Many prominent researchers of the day believed at the time that these problems were not amenable to rigorous analytical treatment. Others, however, disagreed. ONRL leaders began calling upon those who disagreed to see what could be done. To begin the research, they called upon young pioneers in the then, emerging robotics field. One of those called was Dr. S.S. Iyengar. Under the Center for Engineering Systems Advanced Research (CESAR) program. Directed by Dr. Chuck Weisbin and Dr. Jacob Barhen others, he, along with other emerging scientists, was challenged to meet three key goals in developing autonomous robotic systems. The systems needed to be intelligent enough to;
- Make decisions about actual and potential radiation leaks
- Decide on courses of corrective actions through onboard, real-time path planning with sensor feedback and,
- Execute radiation mitigation and clean-up
Prof. Iyengar developed pioneering methods that provided sound analytical and computational basis for developing navigation algorithms in environments with significant unknown parts using the unreliable sensors. Prior to his work, it was unclear if such rigorous frameworks can be developed at all for these complex problems.
Radiation leak decisions
With sponsorship from DOE, Dr. Iyengar worked closely with ORNL team to develop and implement sensor-driven terrain model acquisition and incidental learning methods for mobile robot navigation.
The Hostile Environment Robotics Machine Intelligence Experiment Series (HERMES I, II, III) was developed as a series of experimental mobile robots as testbeds for developing and demonstrating new algorithms for real-time path planning with sensor feedback for decisions for navigating in unstructured environments to determine potential radiation leakage. The HERMIES-IIB robot in the CESAR lab at ORNL is used in research related to vision, multi-sensor integration, coordinated arm movement, path planning, parallel algorithms, and learning. Iyengar also developed a visual object recognition system for HERMIES. This system was implemented on a NCube system, which is an MIMD hyper cube parallel computing system. In general, the input to the object recognition system is an image containing one or more instances of the known different types of obstacles and produces the output location(s) of the obstacle(s) relative to the cameras. Based on assumptions about the known geometry of the obstacles, the viewing conditions, the relevant range of viewing distances and the image resolution needed, the system he developed is able to recognize obstacles by rough, rectangular outlines in the image. The object recognition system used Hough transforms to find rectangular outlines in the image, and then used a sequence of constraints to eliminate those outlines which could not be due to obstacles.
Through a series of works spanning more than a decade, he published a number of pioneering papers in IEEE Transactions on Robotics and Automation and International of Robotics Research, which laid the foundations many of the recent advances in this area. These algorithms discovered in this research have now been advanced and included in nearly all autonomous robots.
6.2 Terrain Mapping Methods
Of particular relevance are the terrain mapping methods he developed, which enabled autonomous robots to cover specific geographic areas. More generally, in this area of computational research, i.e., aspects of robot motion planning in unknown terrains, he is among the first researchers to formulate efficient tractable algorithms and data structures and implement them on practical systems while at ORNL. His work titled “Trajectory Planning of Robot Manipulators in noisy workspaces using Stochastic Automata” published in the International Journal of Robotics Research, Apr 1991 (MIT Press) has been cited extensively by researchers all over the world.
6.3 Market for the Terrain Mapping Technology
Iyengar’s concept has today developed into diverse concepts such as the Roomba vacuuming robots, which are deployed as labor saving devices in households throughout the country. His technology was of decisive importance in developing robots to detect and defuse improvised explosive devices (IED) for use by U.S. military in Iraq and Afghanistan through small, mobile robots. Other recent developments incorporating his technology include self-driving cars using multiple sensors to travel across our highway systems. While the sensors have become much more sophisticated, the origins of the underlying methods can be traced back to his original research discoveries as outlined in his published papers.
6.4 Economic Impact of Sensor-Based Autonomous Mobile Robotics
In a research study published in 2016[1], researchers recently indicated autonomous vehicles and intelligent robots as one of the hottest emerging technologies driving corporate strategies and industry policies. Economically, this has already translated into a more than $640 million per year commercial revenue stream, which is growing at a rate of nearly 10 percent per year.
Detailed References on the Intelligent Mobile Robot
The following articles were published as a part of Dr. Iyengar’s extensive work.
Kai Storjohann & Eric Saltzen, “Adapting Sensory Data for Multiple Robots Performing Spill Cleanup,” Office of Basic Energy Sciences, Oak Ridge National Laboratory, September 1990
This paper describes a possible method of converting a single performing robot algorithm into a multiple performing robot algorithm without the need to modify previously written codes. The algorithm to be converted involves spill detection and clean-up by the HERMIES-III mobile robot. In order to achieve the goal of multiple performing robots with this algorithm, two steps are taken.
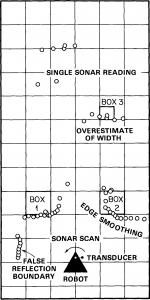
Charles R. Weisbin, “Intelligent-Machine Research at CESAR,” AI Magazine Volume 8, Number 1, 1987
Many technologies of interest to the Department of Energy (DOE) require hazardous operations in which intelligent machines could be used to advantage. Closest to home is the handling of radioactive material around reactors and processing plants, but similar problems arise with explosives and other hazardous chemicals. Underground mining puts humans at risk on a large scale, and underwater operations from exploration to maintenance of equipment and, eventually, to seabed mining have a similar potential.
Deanna L. Barnett, G. de Saussure and F. G. Pin, “Robot Navigation Research at CESAR,” Center for Engineering Systems Advanced Research, Oak Ridge National Laboratory
A considerable amount of work has been reported on the problem of robot navigation in known static terrains. Algorithms have been proposed and implemented to search for an optimum path to goal, taking into account the finite size and shape of the robot. Not as much work has been reported on robot navigation in unknown, unstructured, or dynamic environments.
Edited Books
S. S. Iyengar and A. Elfes, (ed), “Autonomous Mobile Robots: Perception, Mapping, and Navigation,” Volume 1, IEEE Computer Society Press, October 1991.
S. S. Iyengar and A. Elfes, (ed), “Autonomous Mobile Robots: Planning, Control, and Architecture,” Volume 2, IEEE Computer Society Press, October 1991.
Footnotes:
[1] Yun, JinHyo Joseph, DongKyu Won, EuiSeob Jeong, KyungBae Park, JeongHo Yang, and JiYoung Park. "The relationship between technology, business model, and market in autonomous car and intelligent robot industries." Technological Forecasting and Social Change 103 (2016): 142-155.
7. Biomedical Engineering and Medicine
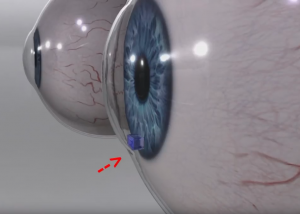
Dr. Ram Iyengar has been elected to the 2017 Class of the College of Fellows of the American Institute for Biological and Medical Engineering. Only 2% of researchers conducting biomedical/bioengineering research worldwide have been inducted with this year only 60% of the nominated candidates made it to the final ballot.
Dr. Iyengar’s innovation and research accomplishments over the last four decades have had a major impact in Computational Sciences with Applications in Medicine, Biological and Medical Imaging. A role model in multiple fields, Dr. Iyengar’s innovations, inventions and commercialization activities have significantly impacted Computer Science, Biomedical Engineering, and Medicine, as well as society as a whole. Dr. Iyengar was a Roy Paul Daniel Professor and Chairman of the Computer Science Department at Louisiana State University for 32 years, where he was responsible for making the LSU computer science program one of the top 10 of 126 colleges and universities in the NRC rankings. He was also the founding Director of the Louisiana Biomedical Research Network.
During his tenure at Oakridge National Lab, he was one of the key leaders responsible for initiating and coordinating a program in autonomous intelligence systems for detecting radiation during the 1980s. He continued his work at the Jet Propulsion Laboratory in the 1990s. He has been a visiting Professor at various institutions, including, the University of Paris, Indian Institute of Science, and other universities in China, Korea, and other universities around the world.
Dr. Iyengar’s inventions which have significantly impacted the field of biomedical engineering and medicine include his newly patented glaucoma detection device. In addition, Dr. Iyengar has been involved in solving complex problems in early detection of lung cancer, by joining colleagues at Southwestern Medical School in Dallas.
7a. Computational Measures for Predicting DNA Mutation
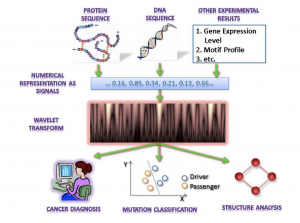
Click to read full article
Dr. Iyengar has developed computational measures for predicting DNA mutations during cancer Evolution, using wavelet analysis in cancer genome research, and designing smart biomarkers for bioremediation.
Juan C Martinez, Nelson L Jimenez, Tao Meng, S.S. Iyengar. “Predicting DNA mutations during cancer evolution.” International Journal of Bioinformatics Research and Applications, Vol. 11, No. 3, pp. 200-218, 2015.
Bio-systems are inherently complex information processing systems. Their physiological complexities limit the formulation and testing of a hypothesis for their behavior. By processing the sequencing data before relapse time, we tested our framework by predicting the regions of the genome to be mutated at relapse time and, later, by comparing our results with the actual regions that showed mutations discovered by genome sequencing at relapse time. This high performance from our proposed framework opens new research opportunities for bioinformatics researchers and clinical doctors.
Soliman AT, Meng T, Chen SC, S. S. Iyengar, Puneeth Iyengar, Yordy J, and Shyu M.L., “Driver Missense Mutation Identification Using Feature Selection and Model Fusion,” Journal of Computational Biology, Vol. 22, No. 13, pp. 1075-1085, Dec. 2015.
Driver mutations propel oncogenesis and occur much less frequently than passenger mutations. The need for automatic and accurate identification of driver mutations has increased dramatically with the exponential growth of mutation data. Current computational solutions to identify driver mutations rely on sequence homology. Here we construct a machine learning–based framework that does not rely on sequence homology or domain knowledge to predict driver missense mutations.
7b. More precise radiotherapy treatment of lung tumors
S.S. Iyengar, Xin Li, Huanhuan Xu, Supratik Mukhopadyay, N. Balakrishna, Amit Sawant and Puneeth Iyengar worked towards providing a better more precise radiotherapy treatment of lung tumor computer in January 2012. Dr. Iyengar began examining the problem of detecting lung cancer through motion tracking, pattern recognition, and high-performance real-time processing. His 4D motion model can now perform 4D monitoring of the entire irradiated anatomical volume, significantly increasing a physician’s confidence in prescribing more aggressive radiation treatments. This is possible since the invention measures more precisely the dosages administered to the patient, thereby assuring the surgeon that dosages are exactly placed to determine treatment efficacy since dosage accuracy is assured. While this treatment is still limited in use, indications are this treatment will significantly increase the lifespans’ for cancer survivors. A computational framework for modeling the respiratory motion of lung tumors provides a 4D parametric representation that tracks, analyzes, and models movement to provide more accurate guidance in the planning and delivery of lung tumor radiotherapy.
7c. Early Glaucoma Detection
Dr. Iyengar and his former colleagues at Nulogix invented a device with an innovative technology that enables a person to monitor and see changes in intra-ocular pressure (IOP) simply by looking at his eye in the mirror. The purpose of this invention is to ensure that glaucoma’s silent damage is detected as early as possible before pressures rise to a level where damage to the eye can occur. It will be especially useful to people diagnosed early and who are at high risk of getting their retinae damaged by increased intra-ocular pressure, such as those with high blood pressure or a genetic history of glaucoma. The device was also developed for those living in rural areas where medical care and the currently large, expensive diagnostic equipment is not available for monitoring daily or weekly changes in the eye’s pressure.
The U.S. Patent Office and the World Intellectual Property Organization have published patent applications filed by Dr. Iyengar and Nulogix for this invention. The company was recently named a finalist in the Enterprise Charlotte Economic Council’s “Innovation 2 Industry Florida” competition for this technology and is seeking investment and clinical partners.
8. NSF I/UCRC Center for Knowledge Enablement
Dr. Iyengar is a Co-Principle Investigator of the NSF Industry-University Cooperative Research Center (I/UCRC) Program, Center for Advanced Knowledge Enablement (CAKE) at FIU.
IUCRC enables industrially-relevant, pre-competitive research via a multi-member, sustained partnerships between industry, academe, and government. NSF supports the development and evolution of IUCRCs, providing a financial and procedural framework for membership and operations in addition to best practices learned over decades of fostering public/private partnerships that provide significant value to the nation, industry and university faculty and students.
The CAKE Center studies the representation, management, storage, analysis, search and social aspects of large and complex data. The research is applicable to biomedical, defense, disaster mitigation, homeland security, environmental concerns, real estate, health records management, finance, and technology service companies. The faculty carries out research in performance studies, benchmark evaluations, and the application of novel algorithms, routines, data models, network analyses and software tools to large-scale data sets. The research being carried out in the universities in this area and more broadly in information technology underpins advances in virtually every other area of science and technology and provides new capacity for economic productivity.
Every two years NSF publishes a Compendium of Technology Breakthroughs of NSF I/URC Centers, a book to advise U.S. Congress and Public on the state of science and technology.
The 2014 NSF Compendium featured on its cover work of the FIU NSF Center: TerraFly and Balloon aerial platform co-sponsored by NSF and Altametry Inc: http://cake.fiu.edu/Compendium-2014/
The 2016 NSF Compendium just published showcases six articles about breakthroughs of our Center, more than any other NSF I/UCRC center in any discipline in the country. The 2016 cover features work led by Dr. Sakhrat Khizroev “Wireless Deep-Brain Stimulation With Magnetoelectric Nanoparticles” at the FIU NSF I/UCRC Center. This work is co-sponsored by NSF and the Neuroscience Centers of Florida Foundation: http://cake.fiu.edu/Compendium-2016/